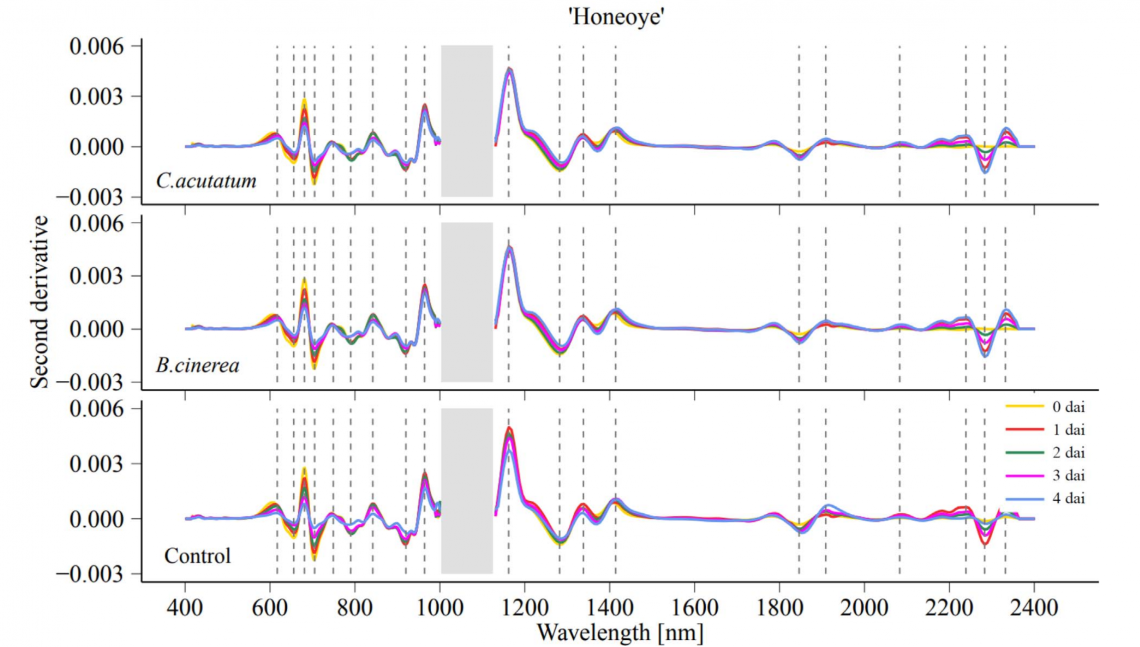
Detection of fungal infections in strawberry fruit by VNIR/SWIR hyperspectral imaging
Anna Siedliska, Piotr Baranowski, Monika Zubik, Wojciech Mazurek, Bożena Sosnowska
Early stages of fungal infections in strawberry fruit are difficult to detect by the majority of commonly used manual and automatic sorting methods In this study, hyperspectral imaging was applied for detection of fungal infections causing fruit spoilage. Fruit of two cultivars: ‘Senga Sengana’ and ‘Honeoye’, were individually inoculated with pathogenic fungi of Botrytis cinerea and Collatotrichum acutatum, while non-inoculated fruit were used as a control. The reflectance spectra of fruit were acquired during four days after inoculation. Based on the second derivative of the original spectra, 19 wavelengths were selected as the most appropriate for discrimination of infections in strawberries and were used to construct supervised classification models. Among all the studied classifiers, the best prediction accuracies for discriminating fungi species were obtained by using the backpropagation neural network (BNN) model. The accuracy of distinguishing between inoculated and control fruit was higher than 97%. The method presented in this study also allowed early detection of fungal infection, starting 24 h from inoculation when the symptoms of fungal infection are invisible. Multiple linear regression was used to create total anthocyanin content (AC), soluble solid content (SSC) and total phenolic content (TPC) models. Two wavelengths (681 and 1292 nm) were selected to create the AC model and four wavelengths (705, 842, 1162 and 2239 nm) were indicated as the most suitable for the SSC model. The coefficients of determination of the created models were R2 = 0.65 and R2 = 0.85 for AC and SSC, respectively. The results demonstrated that hyperspectral imaging technique has potential for rapid and non-invasive detection of fungal infection and for predicting and visualizing AC and SSC in strawberry fruit during storage.